AI Was Big in Davos! (or: How I Learned to Stop Worrying and Love AI)
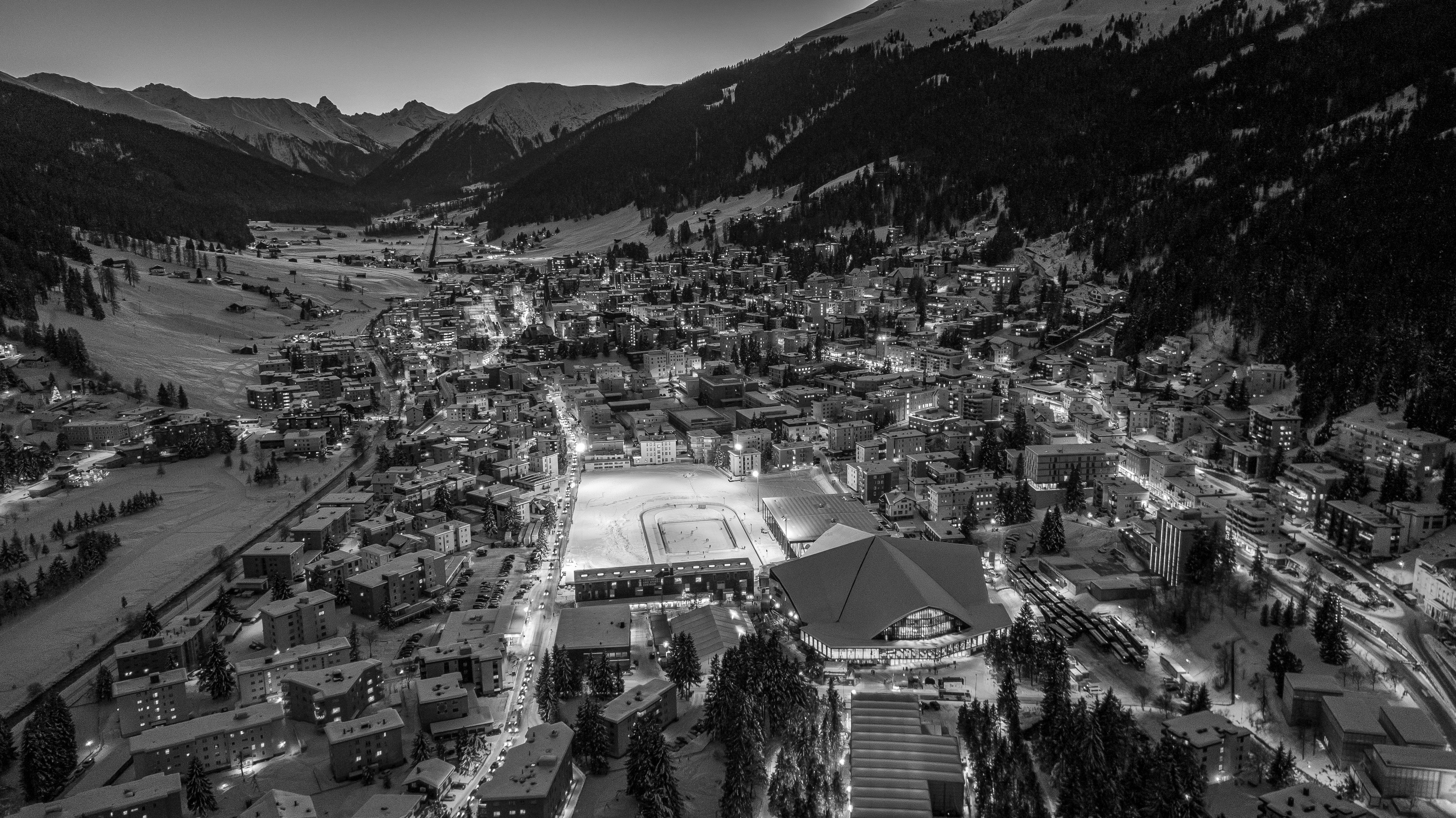
Most in attendance who I spoke to were really looking to understand what it can do today, how it actually works, how much it should all cost, and what the next evolution will be.
We’re at the early internet stages with AI applications – but I think current LLMs are more like newspapers in the 1990s when the internet was just coming online. Many leaders are applying the ‘get in early’ strategy, not unlike Internet 1.0 and the Metaverse after that. I think early adoption is important to get the experience you’ll need to build on, while working toward (and continuing to research) better models. And, of course, ensuring that investments create ROI.
TLDR from Davos: Companies should invest in LLM applications, expensive as they are, in concert with traditional machine learning initiatives to get value from these models and stay in the hunt as the tech improves. Additional AI takeaways from Davos include:
The best application of Generative AI is to solve the “last mile” problem of Data Science and Analytics adoption. This serves to make the tech, and the insights that come with it, accessible to a broader audience.
The future of AI is Agents, ever larger models, better learning, and Robotics (yes, we will see the light on bipedal domestic AI servants). More immediately, LLMs are incredibly expensive as a replacement for traditional ML methods, so the strategy here should be to use LLMs in order to enhance access to techniques and models where their value is established (read ‘the value frontier’).
A lot of large enterprises are selectively deploying LLMs effectively as enhanced search, which is a near-perfect use case for these models. Better, easier access to documentation and internal knowledge bases enhance the effectiveness of existing personnel. These applications with access to public or non-sensitive information can be employee and customer facing and provide basic instructions, policies, and answers to general questions.
LLMs are significantly more expensive to maintain as a replacement for traditional ML applications. (A lot of leaders don’t think about total cost of ownership for ML systems). Per Brad Lightcap, LLMs are the most expensive database systems you can deploy, if you use them that way. However, companies can see real value in LLMs when used to harness more adoption of their existing or newly built machine learning infrastructure, applications, and advanced analytics. This way, they can get more ROI on their existing investments and still be in the game as LLMs and AI, in general, improve. As new models are released, those with experience in these systems will be poised to take advantage of gains in efficiency and accuracy.
GenAI Labs remain the instinctive reaction among the Fortune 50 which are building their own in-house environments for experimentation. Smaller companies are, generally, dipping their toes into ML/AI which are not yet yielding much in the way of results. There’s a critical mass (or killer app) needed to get real traction in these companies which cannot really be attained by hiring more junior talent or asking standing tech leadership to do this work off the proverbial side of their desks. Smart leaders know that the AI race is well underway, and are already working to resource and assemble AI-specific teams that can build and deploy innovative AI features and net new products. I know this has been a big area of focus at Worky of late, as AI hiring demand now rivals ML ops.
Another topic (and shameless plug) in the immediate term, models will continue to get bigger. However, continuous learning language models like Quuery’s Keyword Engine and models that can get more information value per unit of data are the next evolution (and in research labs now). The next (big) jump in AI capability is likely to come from these models, as they can be used by existing LLM applications and also offer extensive insight generation on their own.
Retrieval Augmented Generation (RAGs) are a key part of the LLM deployment strategy. RAGs reduce AI hallucinations (what happens when AIs anticipate what we want to hear and deliver false or misleading information) and provide more timely information to the LLM. In short, this is the current value battleground. Access to high quality proprietary data (a moatable advantage for now) that is easily accessible via vector databases. As more RAGs are deployed, the vector search strategy will become more important; vector similarity is the most common approach, but established models like Quuery’s patented Engine and newer search/similarity models that can extract more info from the data will evolve from these systems.
Good Generative AI deployment strategies, today, are in non-sensitive environments (data sensitivity and low stakes for response error). These can be extended by human-in-the-loop architectures, for example GenAI develops chat responses to customer inquiries, and a human checks/edits the response before release. This yields approximately 4-5X automation for simple applications. Programmers using Gen AI for code perform as if they have 2-3 more years of work experience and students using Gen AI experience an approx 0.25 improvement in GPA.
AI Regulation was among the top concerns at Davos, particularly for Foundation models (Large # of parameter LLMs), but also anywhere an AI would make a significant decision (crime & punishment, credit & lending, housing, medical care, school admission) where biases and/or discrimination could be an issue. This is now in place in the EU, and forthcoming in the US. Also, the fear of a Skynet-like AI was part of the discussion with requirements to be able to “pull the plug” on the rise of new Agents; AIs that can effect change in the world (via APIs). Even for a tech insider and enthusiast like me, this risk cannot be dismissed and must be accounted for.
Common AI Reputational Risks like Sensitive Data Leakage and low Response Accuracy (70-80% when consumers typically demand 99.7%) indicate that chat & user iteration will continue to be necessary for these models to function within acceptable user expectations. This makes defensive data science, i.e. data governance and trust & safety programs a more important part of the strategy when deploying LLMs than with more traditional consumer-facing AI products.
Also for the record, no Gen AI was used to create this content.